Quantum-data-driven dynamical transition in quantum learning
Bingzhi Zhuang, Junyu Liu, Liang Jiang, Quntao Zhuang
Quantum circuits are an essential ingredient of quantum information processing. Parameterized quantum circuits optimized under a specific cost function -- quantum neural networks (QNNs) -- provide a paradigm for achieving quantum advantage in the near term. Understanding QNN training dynamics is crucial for optimizing their performance. In terms of supervised learning tasks such as classification and regression for large datasets, the role of quantum data in QNN training dynamics remains unclear. We reveal a quantum-data-driven dynamical transition, where the target value and data determine the polynomial or exponential convergence of the training. We analytically derive the complete classification of fixed points from the dynamical equation and reveal a comprehensive `phase diagram' featuring seven distinct dynamics. These dynamics originate from a bifurcation transition with multiple codimensions induced by training data, extending the transcritical bifurcation in simple optimization tasks. Furthermore, perturbative analyses identify an exponential convergence class and a polynomial convergence class among the seven dynamics. We provide a non-perturbative theory to explain the transition via generalized restricted Haar ensemble. The analytical results are confirmed with numerical simulations of QNN training and experimental verification on IBM quantum devices. As the QNN training dynamics is determined by the choice of the target value, our findings provide guidance on constructing the cost function to optimize the speed of convergence.
CQN Authors
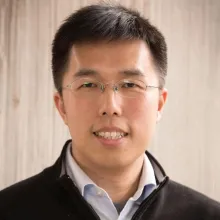
Liang Jiang
Professor of Molecular Engineering
University of Chicago, Pritzker School of Molecular Engineering
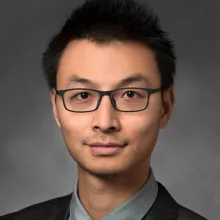
Quntao Zhuang
Assistant Professor
University of Souther California, Ming Hsieh Department of Electrical and Computer Engineering